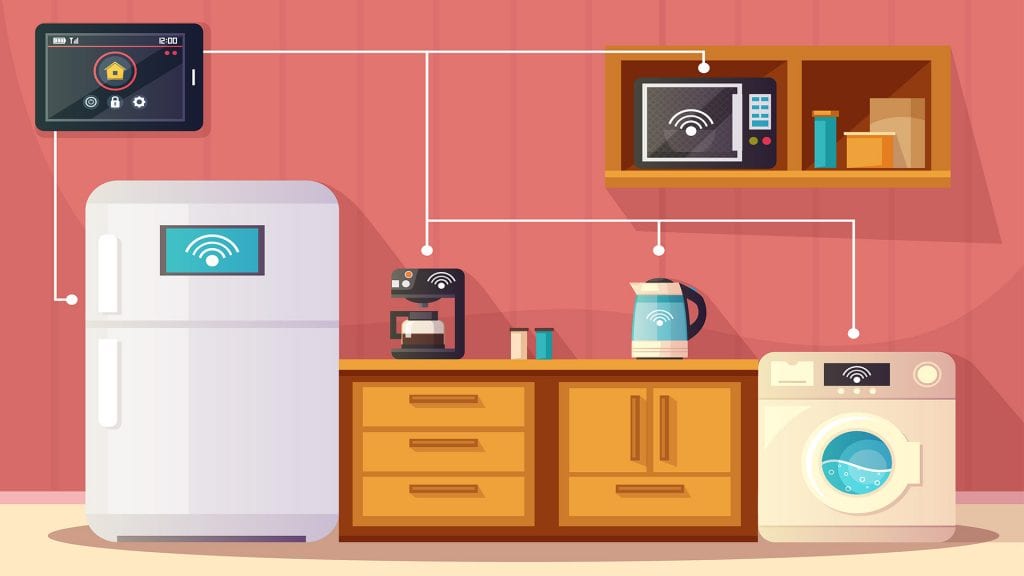
Intel RealSense technology at the Edge
Human Computer Interaction, the edge and the future.
Today, we have a variety of ways that we interact with computers – from buttons and switches in our cars, to keyboards, mice, touchscreens and voice control. Most of the ways we interact with compute are very noticeable to us, and usually they are active – we choose when and how we input information into a system in order to get the results we want.
While this paradigm will still exist in the future, one of the things we are starting to see more of is a more passive and invisible interaction with technology, where much of the processing of data happens on custom processors and chips built into devices. These devices, in some cases, will operate and interact with us in much more opaque ways. In this post we’re going to talk a little bit about IOT, compute at the edge and the future of human computer interaction.
What is the edge?
Today, much of the time we spend online consists of interacting with data and services that live in the cloud. In other words, when you are watching a movie on Netflix, reading social media posts or checking your google mail, most of the hard work is being done somewhere other than your local device. Data is being shuffled to you as needed, but it requires that every device you use has at least the ability to connect to wifi or some other data hungry connection.
When we start talking about the edge, what we really mean is that instead of processing all that data somewhere in a server, some of the interaction might happen locally, on a device that has been specifically designed to process necessary input and output without needing to refer to the cloud.
Depth at the edge
Intel® RealSense™ devices actually fall into this category of edge compute. Whether you are looking at our depth cameras or tracking cameras, they are all utilizing custom designed chips (for example, an Application Specific Integrated Circuit, or ASIC) to process the depth or tracking information. That’s why these devices can run on something as simple as a raspberry PI or a mobile phone without needing anything other than a USB connection to supply some power and to offload the processed data for use. A general processor like an Intel CPU is designed to be able to handle many different tasks with speed and ease. An ASIC like the Intel RealSense Depth ASIC D4 in contrast, has been optimized to do a limited number of calculations but to do this well and efficiently, processing millions of depth points per second at low power.
There are lots of advantages for compute at the edge. In many cases we don’t want or need every device around us to be connected to the cloud, and it’s often much cheaper and faster to have devices able to handle their single use tasks alone. As an example, think about kitchen utensils. A knife is a general purpose tool – it can handle a wide variety of tasks and food types and do so reasonably well. There are times however, when a specialized tool for a job is much more efficient – a melon baller may only have one main use, but it’s a much more effective tool for that one specific job than anything else would be. The same is true of smart devices.
An example use case
Falling is a well known hazard for people of advancing years. Every year, 3 million older people seek hospital treatment for falls. Currently, one of the most popular ‘solutions’ for falling safety is a wearable emergency button. The person has to remember to wear this item every day, keep batteries charged, and then hope that in the event of a fall, it works as intended, and that the person is both conscious enough and able to push the emergency button so that help can be sent.
This is an example of one of the active systems discussed earlier – it requires both remembering to wear the device, and then being actively able to use it when needed – what if the user loses consciousness when they fall, or injures their hands or wrists making pressing the button difficult? What if they land on top of the device and can’t access it – all of which are plausible scenarios.
A different system could utilize depth cameras placed around the house, with small amounts of compute running machine learning models designed to detect falls. The advantage of a system like this is that it would be passive and not require any external monitoring or for any of the camera information to leave the device to be processed elsewhere, hopefully assuaging privacy concerns. Depth cameras running a skeletal tracking system could easily identify a person falling and lying on the floor, and all of this could happen at the edge. No videos of someone in their house would leave the local devices, the depth cameras simply functioning as a sensor to detect falls accurately, rather than as a camera as such.
A fall detection system utilizing the depth cameras and edge compute in this way would be able to run and react quickly regardless of home internet speeds – the only time the devices would need to interact with the outside world would be in the event of a fall, to automatically contact help. The advantage of the edge in a use case like this is clear.
Human computer interaction in the future.
As we can see from the example above, in this case, how the user interacts with a system like this is passive, something we will see more of as smarter, more efficient and accurate devices enter our homes. Imagine light switches as a thing of the past, where rooms light up as you enter them and turn off as you leave. Doors that unlock as you approach, and lock when you leave. Computer vision devices like depth cameras allow this kind of passive interaction to become ubiquitous – universal sensors that can understand what we want and need before we do.
Subscribe here to get blog and news updates.
You may also be interested in
In a three-dimensional world, we still spend much of our time creating and consuming two-dimensional content. Most of the screens
A huge variety of package shapes, sizes, weights and colors pass through today’s e-commerce fulfilment or warehouse distribution centers. Using
Let’s talk about how Intel RealSense computer vision products can enhance your solution.