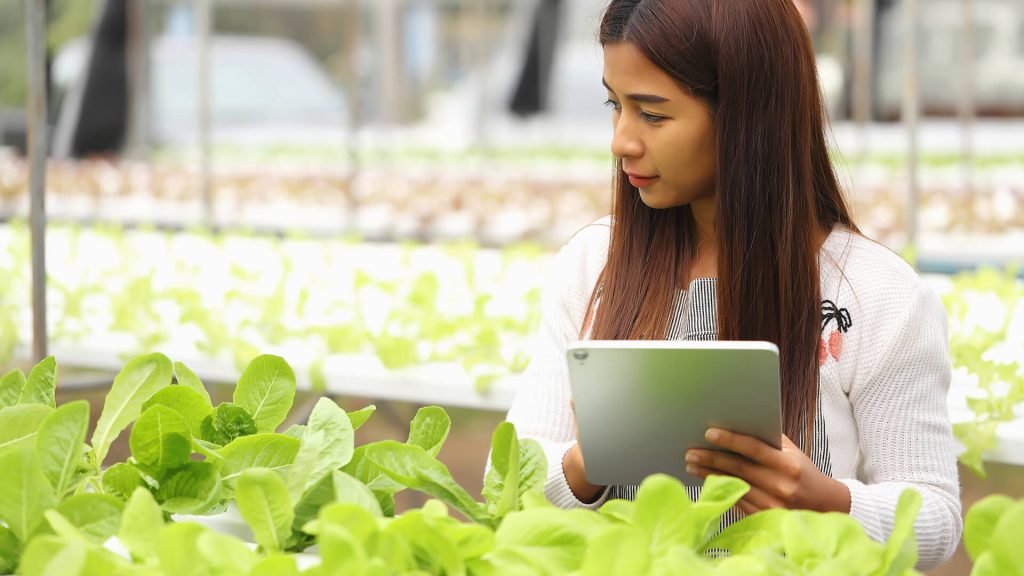
Depth and food: data driven farming
Data is important. Whatever it is you are studying, it’s crucial to have as much, high quality and accurate data as possible. When it comes to food production and farming, there are so many different variables that collecting quality data is crucial to improve production, reduce waste, and improve quality and flavor. With challenges from climate change, increasing population, urban sprawl and more, farming is an industry where analytics can make a massive difference. In this blog, we will look at a number of academic agricultural studies utilizing Intel® RealSense™ Depth cameras to collect quality data.
Plant factories and lighting
Many variables affect crop growth, from environmental factors like sunlight and rainfall, to pests, soil nutrients and more. In order to control this environment more effectively and maximize stable supply of agricultural products, plant factories are becoming increasingly popular. These are large commercial greenhouses where every aspect of the plant’s growing environment is completely controlled. This includes using fluorescent lamps, LEDs and recently Light Emitting Plasma (LEP). LEP differs from other methods because it has a continuous spectrum of light similar to that of sunlight. While empirically it has been shown that LEP lighting can achieve better plant growth when compared with LED lighting, quantitative measurements that show the relative difference between these two lighting methods has not been performed.
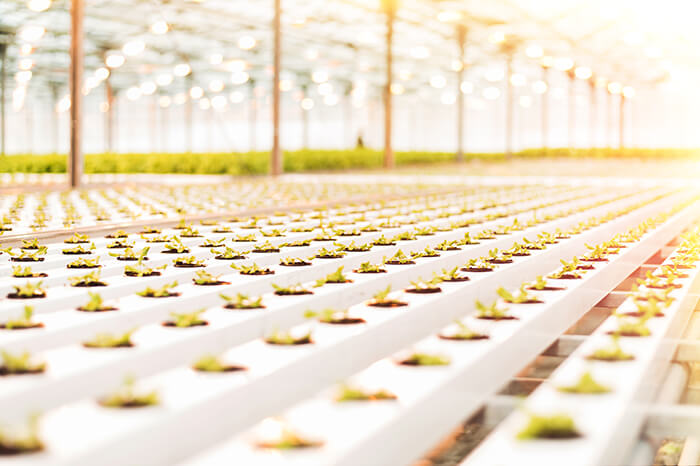
Commercial Greenhouse
To fully optimize plant growth, quality data is needed. A conventional 2d camera cannot easily be used to measure geometric data such as stem height, leaf area and fruit volume, so in pursuit of better data, this study looked at using an Intel RealSense depth camera to accurately evaluate plant growth in different lighting conditions.
In this case, plants were placed on a turntable which was then rotated at constant speed, while the camera captured point cloud data for the plant in one degree increments. Since the plane of the turntable surface is known, finding polygons with a normal (that is a line that is 90 degree direction from the surface of the polygon) similar to the turntable, the surface of the soil can be determined. This baseline of the soil can then be used to measure stem height. Similarly, surface normals were used to calculate leaf sizes.
This method consistently showed a negligible (~1%) difference when compared to manual measurements with a ruler, demonstrating that this system can be used to evaluate plants in a variety of conditions. It could also be possible to mount such a system on a robotic arm, allowing for evaluation of plants in situ in a plant factory.
Fruit, flower or leaf?
Accurately identifying different parts of plants is important for autonomous harvesting solutions. It’s also important to allow accurate yield prediction. The number of flowers directly correlates to the number of fruits harvested for example. In this paper the goal is to accurately detect yellow tomato flowers from images taken in a production greenhouse. With restrictions on where the cameras can be placed, variation in orientation, overlap and occlusion, this is a hard problem to tackle.
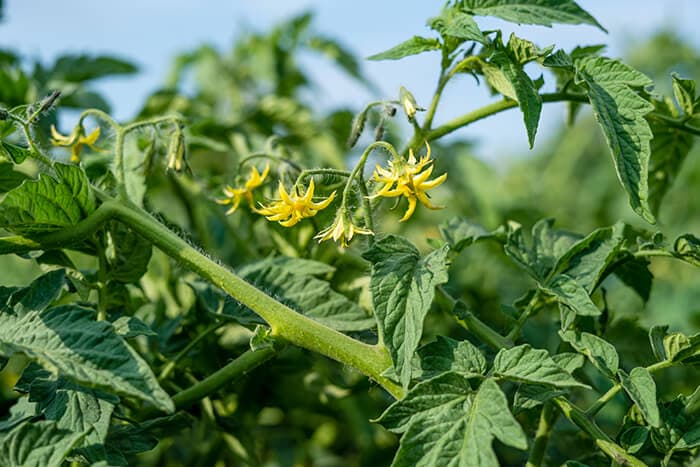
Flowering tomato plants
Various other studies have tried to solve this problem, using drones, high definition cameras and a number of different convolutional neural network or machine learning methodologies to identify flowers. In this case, by using an Intel RealSense depth camera D435, the team were able to use the RGB camera to identify the yellow flowers, and intend to use the depth data from the camera in the future to assist with background segmentation and allow the generalization of their model to different lighting conditions, something that would not be possible with traditional 2D cameras.
Are there alternatives to bees?
About 35 percent of the world’s food crops depend on animal pollinators, primarily bees, to reproduce. In recent years however, Colony Collapse Disorder (CCD) has resulted in a shortage of bees for commercial pollination operations. Plant factories that grow within greenhouses also present a problem for bees. Since bees use ultraviolent rays to navigate, greenhouses that limit the wavelengths of light available to only those which are necessary for plant growth can sometimes provide less than effective pollination.
It is possible to manually pollinate flowers, using swabs or brushes to transfer the pollen to the correct area of the plant. In commercial operations this is generally only possible on a smaller scale or is used when there are other reasons to hand pollinate due to the specific species. Since this is labor intensive, it’s not a practical solution for large scale operations.
In pursuit of a better solution, the authors of this paper use an ultrasonic focusing device to vibrate flowers and pollinate by ultrasonic waves. This device was designed as a ‘tactile display’ to generate working force without physical contact. It utilizes multiple ultrasonic transducers that are then focused to generate force in one specific area. This focal point can be adjusted to any point in 3d space.
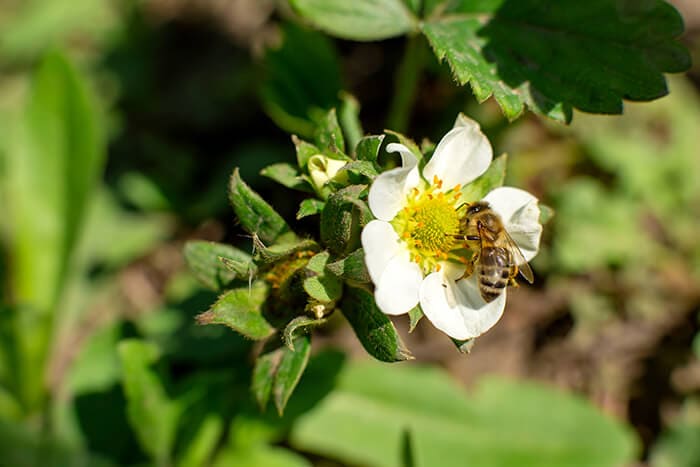
A bee pollinating a strawberry flower
In order to accurately identify the correct location of the flower to vibrate, the team used an Intel RealSense depth camera SR305. By combining the depth camera with the ultrasonic device, they were able to first use machine learning with the RGB output from the camera to identify areas containing a strawberry flower. By setting a boundary size in pixels for the size of the flower, and simultaneously setting a distance parameter, non-flower and background areas were identified and removed, allowing the flower areas to be targeted accurately with the ultrasonic focusing device.
As a part of the test, the frequency of ultrasonic waves was varied between different plants, as well as control plants with either manual brush pollination or no treatment at all was measured. The results showed that a frequency of 40Hz showed the best result, yielding significantly more fruit than other frequencies or the other methods investigated. This suggests that ultrasonic pollination could potentially be a commercial solution for pollination comparable to that of bee pollination treatment at least for some types of crops.
Subscribe here to get blog and news updates.
You may also be interested in
“Intel RealSense acts as the eyes of the system, feeding real-world data to the AI brain that powers the MR
In a three-dimensional world, we still spend much of our time creating and consuming two-dimensional content. Most of the screens